
More analytically rigorous methods for inferring these taxonomic interactions have recently been developed to resolve the biologically relevant interactions and to account for unique statistical features of microbiome data ( Dohlman and Shen, 2019).
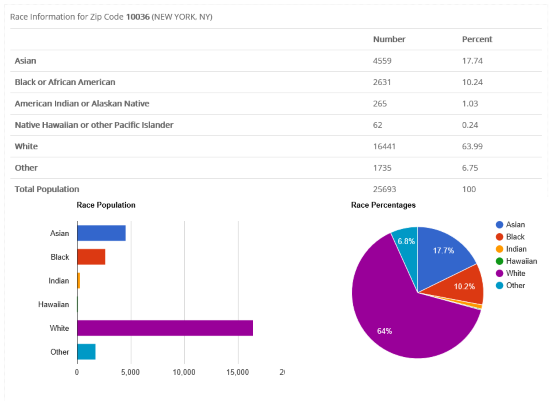
Networks can then be visualized or analyzed using a variety of techniques to resolve, for example, taxa that potentially co-depend on one another, taxa that potentially compete with one another, or keystone taxa ( Faust and Raes, 2012 Layeghifard et al., 2017). These networks have been most widely applied to microbiome taxonomic data and are traditionally assembled by correlating microbiome features and establishing linkages between features based on the significance or magnitudes of these correlations ( Faust and Raes, 2012 Röttjers and Faust, 2018). As a result, they offer a mapping of how information flows among the members of the microbiome or its environment ( Röttjers and Faust, 2018). Networks can be used to itemize interactions between community members, between communities, and between community members and some set of covariates ( Follows et al., 2007 Faust et al., 2012 Gaulke et al., 2016 Tapio et al., 2017 Gould et al., 2018 Mandakovic et al., 2018). To disentangle these mechanisms among the complex milieu of microbiome features, researchers have developed a rich array of analytical procedures, with one of the most widely used being microbiome network reconstruction. One common goal is to resolve how the microbiome influences or responds to its environment ( Alivisatos et al., 2015 Blaser et al., 2016). However, the complexity of microbial systems, which frequently include diverse taxa and ecological covariates, continues to challenge the discovery of biological signal in these massive data sets. By coupling environmental DNA sequencing procedures with bioinformatic and data analytic approaches, scientists have begun to disentangle the composition, diversity, and function of microbiomes ( The Human Microbiome Project Consortium, 2012 Sunagawa et al., 2015 Thompson et al., 2017). The microbiological sciences have undergone a research transformation in recent years as extensive volumes of microbiome data have been generated. We also highlight on-going statistical challenges and opportunities for integrative network analysis of microbiome data. We compare advantages and disadvantages of various statistical tools, assess their applicability to microbiome data, and discuss their biological interpretability. In this review, we overview some of the most important network methods for integrative analysis, with an emphasis on methods that have been applied or have great potential to be applied to the analysis of multi-omics integration of microbiome data. While network analysis has emerged as a powerful approach to modeling microbiome data, oftentimes by integrating these data with other types of omics data to discern their functional linkages, it is not always evident if the statistical details of the approach being applied are consistent with the assumptions of microbiome data or how they impact data interpretation. This emergent analytical toolset frequently ports over techniques developed in other multi-omics investigations, especially the growing array of statistical and computational techniques for integrating and representing data through networks. However, the analytical methodology needed to model microbiome data and integrate them with other data constructs remains nascent. The advent of large-scale microbiome studies affords newfound analytical opportunities to understand how these communities of microbes operate and relate to their environment.
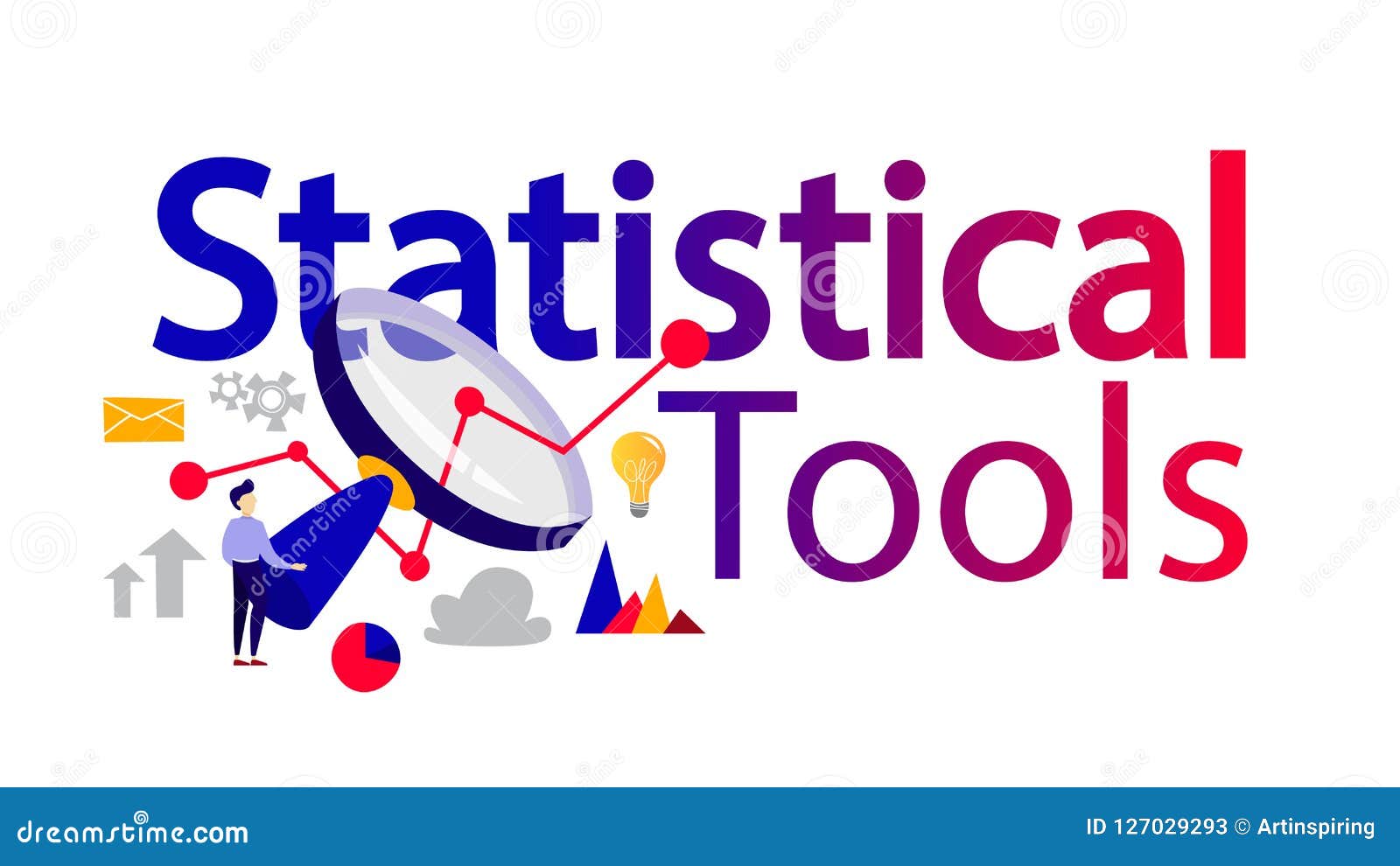
2Department of Microbiology, Oregon State University, Corvallis, OR, United States.1Department of Statistics, Oregon State University, Corvallis, OR, United States.Armour 2, Chenxiao Hu 1, Meng Mei 1, Chuan Tian 1, Thomas J.
